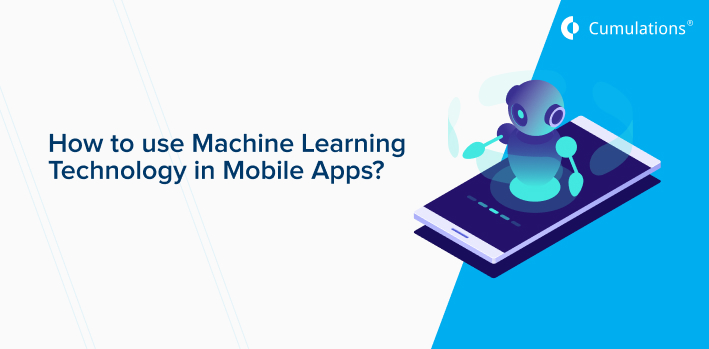
In recent times Machine learning continues to transform a growing array of sectors as a subfield of artificial intelligence. Machine learning (ML) helps consumers to gain insights such as spotting credit-card fraud, improving development procedures, anticipating the behavior of their clients and personal preferences of Web-users using algorithms which are continuously learned from results. This begs the issue of whether machines can learn from previous interactions automatically.
Machine Learning Technology predictions can guide intelligent action without human intervention because of their high accuracy. The development of machine learning apps makes a mobile app smarter. It also means that tasks without a special schedule are completed.
Using machine learning technology in mobile apps
In comparison, the emergence of machine learning is responsible for changing the model of software growth. Application developers that have written such algorithms will never be clear on the intended use and results if the value of the data is not obvious. But machine learning systems now require those to respond to that as well. It helps to capture, organize and archive the correct data into structures so that these patterns can be acquired over time and modified, if possible. Algorithms and data sets are trained using AI techniques. We improve inferior performance in the production of computer apps:
precise tests, all available knowledge will be used
When a basic approach is used, the machine learning algorithm is efficient
Predictability depends entirely on the validity of information
Always check ML algorithms
The impact of machine learning in the creation of applications
With the increase in data analysis mainly automated on analytical model building, many new techniques were introduced. These automated analytical models are suitable for increasing more functions and transforming the development process. Most of the enterprises use data mining to generate new information for the huge data. While the impact on this new way of learning gives more understanding with creativity and saves more time in the process. Enterprises can use Machine Learning Technology as an alternative for conventional data mining. The main objective of machine learning is, therefore, the user-friendly mobile framework.
To fulfil the customer’s needs, one would also stick to the basic rules:
A positive thing is an independent approach. Each consumer needs from an interface the ease and convenience. In reality, any app will become a friend to you using machine learning, who is willing to predict your desires and who can suggest appropriate content in the long run.
The quest will not take time and be challenging. To those who want to find details, ML tools may be useful. Such resources examine the context of the user and standard behaviour; include correction of pronunciation, expression check and a list of relevant demands.
Users enjoy e-commerce features that are optimised. Fortunately, this type of process is perfectly appropriate for machine learning. Furthermore, the customer is likely to get valuable information if data on clicking and transaction levels and search history and shopping habits are available.
The ML algorithm just forecasts your search queries. This helps you to propose the right items, services, platforms and shipping times that meet consumer requirements.
The more details you evaluate, the more you are aware of the needs of our customers. By supplying usage info, you increase the probability of ML being used enormously
Using machine learning in a mobile app
Machine Learning (ML) is an automatic data processing and algorithm innovation for decision-making. These methodologies are intended to improve their procedure based on their results. In general, it means “learning on the go.” The more skilled the ML data, the more precise the ML algorithm.
The three main techniques are used to develop a classifier that unveils links to artificial intelligence
With more modern web applications using this advanced algorithm, it is easier to understand the customer behaviour as well as boosting the customer engagement.
Convenient and simple ways to meet their needs are sought by users. Thankfully, ML Software Design involves forecasting software without performing custom predictions. AI not only offers business incentives, but it also helps companies to address customer questions even quicker, primarily via mobile apps. Therefore, industry leaders use ML in their goods at present,
Learning monitored. when an algorithm learns from example information and related target responses. The data could include numerical values or labels of strings, for example, classes or tags. Machine Learning Technology may subsequently predict the correct answer with new instances.
Learning Uncontrolled. Without any corresponding reaction, ML learns from examples. The algorithm, therefore, determines the patterns of the data by itself.
Learning in enhancement. ML is qualified to make environmental decisions. This allows the computer to correctly take decisions with the best expertise.
TV – Netflix
Most users learn what the Recommendation Program reveals at Netflix. This ensures that ML algorithms rely on the quality of consumers who would not have selected at first. The recommendations are focused on overt and implicit evidence. The first involves the ranking specifically and the other is the evidence on consumer behaviour. Also, Android users are now able to download content on a microSD card and store it per device for 30 days, as ML algorithms are also considered explicit.
Filters for Snapchat
The advanced facial recognition system scans a gazillion faces so that a face with all the features can be identified. Then it will add filters, lenses and goggles, assisted by enhanced reality technologies properly, from the camera facing the smartphone.
Google maps
Predict parking by using machine learning magic. It measures the parking problem using geo-data from users for training models. This tool now covers 25 cities within and 25 non-US cities.
Fiscal taxis
The prediction has been accomplished by the application of modelling technologies and ML. The device refers to the actual position of the driver, the time of the request and history. It gives a smartphone customer with context-conscious destination recommendations. The service makes suggestions for new customers using aggregate information on popular sites.
Bankrolling
Financial machine learning forecasts upcoming patterns, bubbles and crashes. For example, ML can analyze credit rating information about borrowers. For process automation, artificial learning is also useful. What can be used in finance for machine learning? ML eliminates work by hand, increases flexibility, and streamlines routine tasks.
As a result, machine training for application areas in finance has the following advantages:
Reduction by automated processes of capital expenses.
More revenue due to improved user experience and productivity
Improved enforcement and strengthened protection.
Medicine
Medical diagnosis is another area of use for machine learning. Machine learning combines the doctor’s knowledge and increases the efficiency and reliability of treatment in a health system. For diagnostics, custom medicine and other fields where time matters, the ML algorithm is used. The most critical examples involving the use of the healthcare system are:
Diagnose of Heart Disease
Detection and forecast of cancer
Care tailored
Conclusion
Techniques are a secret game changer for machine learning. However, Machine Learning Technology in smartphone apps is designed to construct practical and customized interactions. Such applications will also provide their users with the necessary functionality and services that fuel creativity in all industries. The user and the smart system interact to improve the accuracy of the system.